Delivering Impact from AI in Research, Development, and Innovation
Artificial intelligence (AI) is transforming research, development, and innovation (R&D&I), unlocking new possibilities to address some of the world’s most pressing challenges, including sustainability, healthcare, climate change, and food and energy security, as well as helping organizations to innovate better and launch breakthrough products and services. AI in R&D&I is not new. However, the rise […] The post Delivering Impact from AI in Research, Development, and Innovation appeared first on Unite.AI.
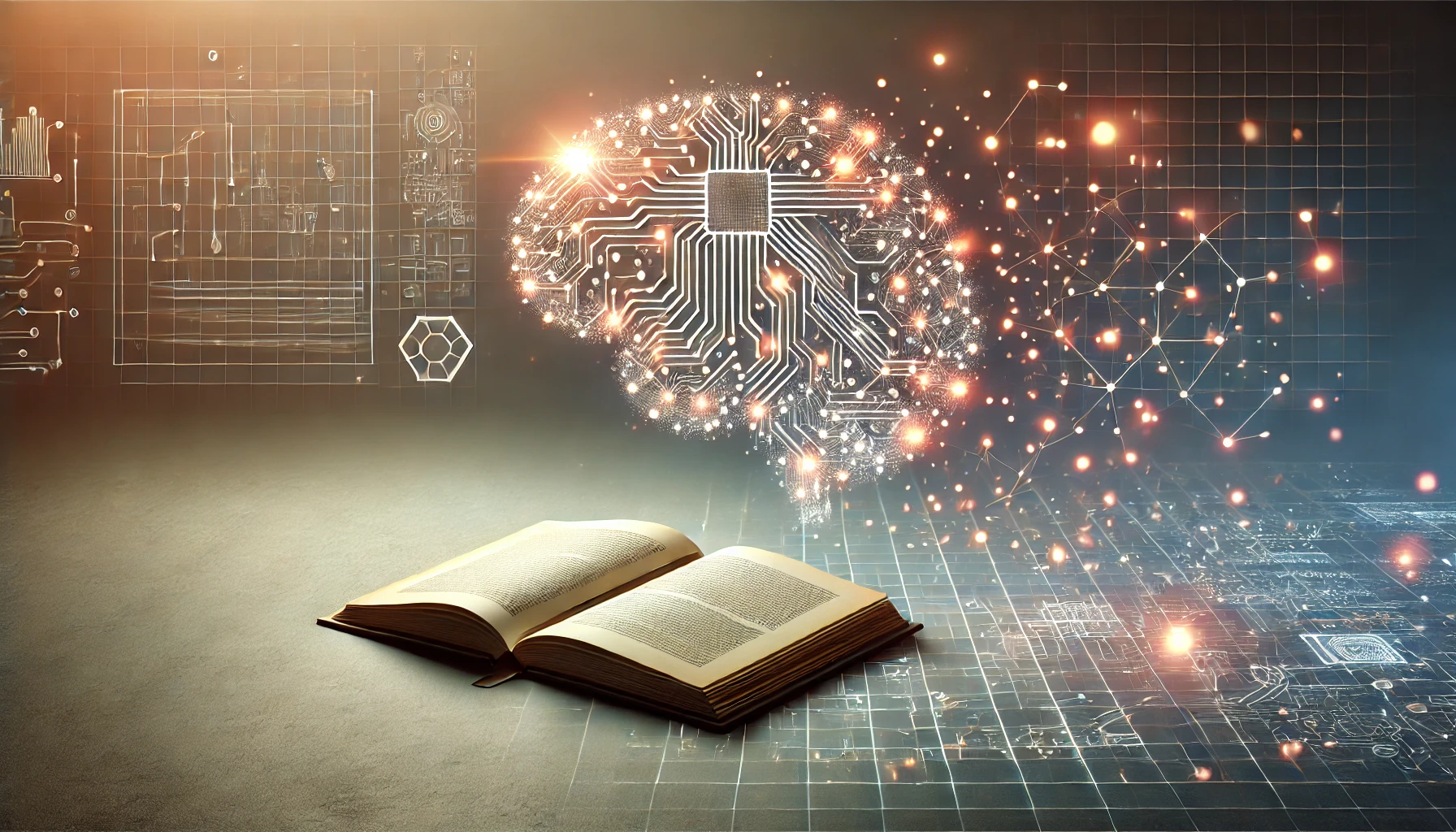

Artificial intelligence (AI) is transforming research, development, and innovation (R&D&I), unlocking new possibilities to address some of the world’s most pressing challenges, including sustainability, healthcare, climate change, and food and energy security, as well as helping organizations to innovate better and launch breakthrough products and services.
AI in R&D&I is not new. However, the rise of generative AI (GenAI) and large language models (LLMs) has significantly amplified its capabilities, accelerating breakthroughs and overall innovation.
How can organizations benefit from AI in their R&D&I efforts, and what are the best practices to adopt to drive success? To find out Arthur D. Little’s (ADL’s) Blue Shift Institute carried out a comprehensive study interviewing over 40 AI providers, experts, and practitioners, as well as surveying over 200 organizations across the public and private sectors. The resulting report, Eureka! on Steroids: AI-driven Research, Development, and Innovation, offers an in-depth analysis of the current landscape and future trajectory of AI in research and innovation.
Our analysis focuses on five key areas:
AI delivers benefits across R&D&I – but it won’t replace humans
Every building block of R&D&I can benefit from AI, from technology and market intelligence to innovation strategy, ideation, portfolio and project management, and IP management. When we look to understand these benefits, three key factors emerge:
- AI will augment researchers, rather than replacing them, freeing up their time, and enabling them to be more productive and creative
- AI helps solve intractable problems that couldn’t be attempted before because of the technology’s speed and ability to scale and learn, opening up new avenues of innovation
- AI will assume a “planner-thinker” position, moving beyond content generation and search to cover more complex roles such as becoming a knowledge manager, hypothesis generator, and assistant to R&D&I teams.
When deciding whether to use AI to solve a specific R&D&I use case there is no blanket model to deploy. To understand which AI approach will give the best results organizations need to focus on two factors – the type and amount of data available (from a little to a lot) and the nature of the question being asked (from open to specific). At the same time, a single AI approach may not deliver optimal results — most state-of-the-art intelligent systems produced in the past 15 years have been systems of systems. These are independent AI systems, models, or algorithms designed for specific tasks, which, when combined, offer greater functionality and performance.
Success requires eight good practices
Based on interviews with researchers, AI scientists, founders, and heads of R&D in digital, manufacturing, marketing, and R&D teams we see eight good practices that underpin successful AI deployment. Organizations need to:
- Adopt agile methodologies so that teams can work quickly in a fast-changing AI environment
- Build robust foundations by focusing on data quality, collaboration across the organization and leveraging proprietary data
- Make a strategic choice between building, buying and fine-tuning models, with the latter approach often the most effective
- Consider analytical trade-offs to ensure progress during proof-of-concept projects, such as around acquiring versus synthesizing data, precision versus recall, and underfitting versus overfitting
- Be proactive in leveraging available data science talent, including partnering outside the organization to acquire necessary skills
- Align with IT to balance security and compliance with experimentation speed
- Demonstrate benefits quickly and get user buy-in to build trust and unlock further investment
- Maintain and monitor system performance continuously, particularly around model improvements
3. The technology components are now in place
As with most AI use cases, the R&D&I value chain comprises three layers – infrastructure, model developers and applications.
In terms of infrastructure, the cost of implementing and maintaining sufficient computing power is large, but hosting providers are increasingly offering inference-as-a-service models, running inferences and queries in the cloud to remove the need for in-house infrastructure, lowering up-front expenses and democratizing access to AI.
The value chain for AI in R&D&I heavily relies on major open source models from players such as Meta, Microsoft, and Nvidia. However, smaller players, such as Mistral and Cohere, also form a key part of the ecosystem, as do academic institutions.
At the application end of the chain, general and specialist R&D&I apps have already been created to meet most use cases, with over 500 now available, covering the entire R&D&I process.
The future is unclear – but scenario planning helps understanding
How AI in R&D&I will evolve depends on the outcomes of three main factors – performance, trust, and affordability. Combining these factors leads to six plausible future scenarios on a spectrum between AI transforming every aspect of R&D&I to being used only in selective, low risk use cases. On a scale from maximum to minimum impact, these scenarios are:
- Blockbuster: AI becomes top of mind throughout the R&D cycle, reshaping organisations along the way. Data becomes the new frontier.
- Crowd-Pleaser: AI is convenient, affordable, and adopted for daily productivity tasks but fails short of delivering scientific/creative value.
- Crown Jewel: AI delivers productivity and scientific breakthroughs, but only to those organisations that can afford it – leading to a two-speed world in R&D&I.
- Problem Child: Despite some hallmark use cases and affordable solutions, AI fails to demonstrate its value – R&D&I organisations remain concerned about data security, deontology, and lack of interpretability.
- Best-Kept Secret: AI performance improves, but high costs make organisations more risk-averse. Low trust and red tape limit adoption with few new bold experiments launched.
- Cheap & Nasty: AI is broadly used in low stakes use cases, but only as a prototyping or brainstorming tool. Untrustworthy systems are strictly vetted, and outputs are verified, curtailing productivity gains.
Understanding these scenarios is important for R&D&I organisations as they chart a way forward for their AI adoption.
The time for R&D&I organizations to act is now
In some situations, AI is already enabling double-digit improvements in time, costs, and efficiency in formulation, product development, intelligence, and other R&D&I tasks. That means no matter which scenario plays out, six no-regret moves will help R&D&I organizations build resilience and leverage the benefits of AI. They need to:
- Manage and empower talent, ensuring the workforce has the training and expertise to harness AI, if necessary subcontracting implementations to external providers in the medium term
- Control AI-generated content, updating risk management processes and sharing validation methodologies publicly to build trust
- Build up data sharing and collaboration, working with the wider public and private sector ecosystem to drive successful AI adoption
- Train for the long run, educating the widest possible user population on both AI fundamentals, required skills, and potential risks
- Rethink organization and governance, moving it beyond IT to give a senior level focus and break down silos to smooth collaboration
- Mutualize compute resources, working with partners or sharing resources internally to cost-effectively meet current and future infrastructure needs
Beyond these no-regret moves, success will come from creating a balanced portfolio of AI-based R&D&I investments aligned with corporate objectives. This means considering the scope, costs and benefits of specific AI use cases and using this to drive optimization of the innovation project portfolio. Decisions should be based on strategic objectives, capabilities, and market intelligence, and the context in which organizations operate.
Every stage of the research, development, and innovation value chain can potentially be transformed through AI, augmenting human researchers to transform productivity and enable breakthrough innovation. These opportunities need to be balanced against a range of challenges around performance, trust, and affordability, meaning organizations must focus now to position their R&D&I AI efforts in order to deliver success, whatever the future brings.
This article was written with the assistance of Albert Meige, Zoe Huczok, Arnaud Siraudin, and Arthur D. Little.
The post Delivering Impact from AI in Research, Development, and Innovation appeared first on Unite.AI.