"Revolutionizing Systems Engineering: The Role of AI in Requirements Analysis"
In the rapidly evolving landscape of technology, systems engineering stands at a pivotal crossroads, where traditional methodologies meet groundbreaking innovations. Are you grappling with the complexities of requirements analysis in your projects? Do you find yourself buried under an avalanche of data and struggling to extract meaningful insights? You’re not alone. Many professionals face these daunting challenges as they strive for efficiency and precision in their work. Enter artificial intelligence—a game-changer that promises to revolutionize how we approach requirements gathering and analysis. In this blog post, we will explore the foundational elements of systems engineering while illuminating the critical role AI plays in transforming this process from tedious to streamlined. Discover how cutting-edge algorithms can sift through vast amounts of information, identify patterns, and enhance decision-making like never before. We’ll delve into real-world case studies showcasing AI’s remarkable impact on successful project outcomes, alongside potential hurdles that may arise during integration. Join us as we navigate future trends poised to redefine our industry—your roadmap to mastering requirements analysis awaits! Understanding Systems Engineering Basics Systems engineering is a multidisciplinary approach that focuses on the design, integration, and management of complex systems throughout their life cycles. It emphasizes understanding system requirements—both functional and non-functional—to ensure product quality and precision. Key components include defining system architecture, assessing performance metrics, and managing risks associated with project development. A robust framework for systems engineering incorporates methodologies such as model-based systems engineering (MBSE) to enhance communication among stakeholders. The Role of AI in Systems Engineering Generative AI plays a transformative role in automating critical steps within systems engineering by leveraging natural language processing (NLP) and machine learning (ML). These technologies facilitate the analysis of requirements based on established criteria from organizations like INCOSE. By classifying requirements efficiently, AI can help identify potential misinterpretations early in the process while improving overall accuracy. As engineers increasingly integrate these advanced tools into their workflows, it becomes essential to evaluate AI models rigorously to ensure they meet industry standards for reliability and effectiveness. Advancements in Computational Techniques Recent advancements also highlight computationally efficient algorithms like Gaussian Process Regression used for solving inverse problems related to linear partial differential equations (PDEs). This method demonstrates high accuracy even amidst noisy data conditions—a crucial factor when modeling real-world scenarios where uncertainty is prevalent. Such innovations not only streamline processes but also pave the way for future applications across various domains within systems engineering. The Importance of Requirements Analysis Requirements analysis is a critical step in systems engineering, serving as the foundation for product quality and precision. By meticulously analyzing system requirements based on INCOSE's criteria, engineers can identify both functional and non-functional needs that drive project success. This process not only clarifies stakeholder expectations but also mitigates risks associated with misinterpretations—especially relevant when integrating generative AI technologies. Enhancing Product Quality Effective requirements analysis ensures that all necessary specifications are captured accurately, which directly influences the final product's performance and reliability. In projects like developing RFID-based systems for managing medical equipment, precise requirement classification becomes essential to meet operational standards. Moreover, ongoing evaluation of AI models used in this context helps refine their capabilities to handle complex engineering demands effectively. Incorporating natural language processing (NLP) and machine learning (ML) techniques into requirements analysis further enhances efficiency by automating classification tasks. However, it remains vital to address potential challenges posed by AI misinterpretations through rigorous testing and validation processes. As technology evolves, so too must our approach to understanding and implementing robust requirements analysis methodologies within systems engineering frameworks. How AI Transforms Requirements Gathering AI is revolutionizing requirements gathering in systems engineering by leveraging Natural Language Processing (NLP) and Machine Learning (ML) techniques. These technologies automate the analysis and classification of system requirements, enhancing both product quality and precision. By categorizing requirements into functional or non-functional types,
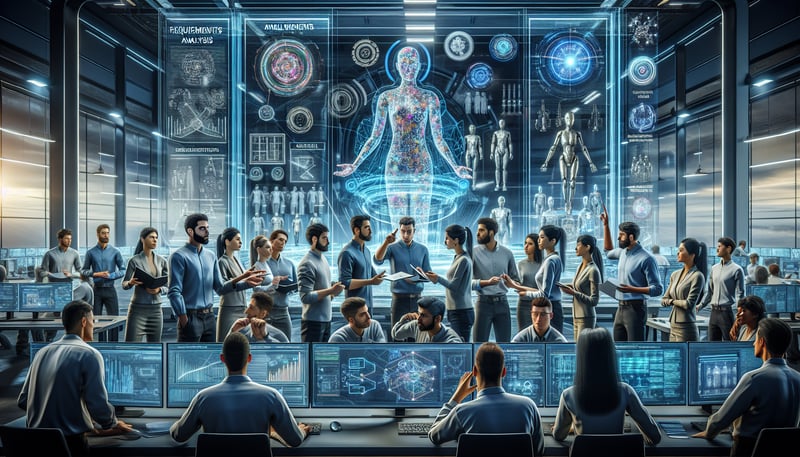
In the rapidly evolving landscape of technology, systems engineering stands at a pivotal crossroads, where traditional methodologies meet groundbreaking innovations. Are you grappling with the complexities of requirements analysis in your projects? Do you find yourself buried under an avalanche of data and struggling to extract meaningful insights? You’re not alone. Many professionals face these daunting challenges as they strive for efficiency and precision in their work. Enter artificial intelligence—a game-changer that promises to revolutionize how we approach requirements gathering and analysis. In this blog post, we will explore the foundational elements of systems engineering while illuminating the critical role AI plays in transforming this process from tedious to streamlined. Discover how cutting-edge algorithms can sift through vast amounts of information, identify patterns, and enhance decision-making like never before. We’ll delve into real-world case studies showcasing AI’s remarkable impact on successful project outcomes, alongside potential hurdles that may arise during integration. Join us as we navigate future trends poised to redefine our industry—your roadmap to mastering requirements analysis awaits!
Understanding Systems Engineering Basics
Systems engineering is a multidisciplinary approach that focuses on the design, integration, and management of complex systems throughout their life cycles. It emphasizes understanding system requirements—both functional and non-functional—to ensure product quality and precision. Key components include defining system architecture, assessing performance metrics, and managing risks associated with project development. A robust framework for systems engineering incorporates methodologies such as model-based systems engineering (MBSE) to enhance communication among stakeholders.
The Role of AI in Systems Engineering
Generative AI plays a transformative role in automating critical steps within systems engineering by leveraging natural language processing (NLP) and machine learning (ML). These technologies facilitate the analysis of requirements based on established criteria from organizations like INCOSE. By classifying requirements efficiently, AI can help identify potential misinterpretations early in the process while improving overall accuracy. As engineers increasingly integrate these advanced tools into their workflows, it becomes essential to evaluate AI models rigorously to ensure they meet industry standards for reliability and effectiveness.
Advancements in Computational Techniques
Recent advancements also highlight computationally efficient algorithms like Gaussian Process Regression used for solving inverse problems related to linear partial differential equations (PDEs). This method demonstrates high accuracy even amidst noisy data conditions—a crucial factor when modeling real-world scenarios where uncertainty is prevalent. Such innovations not only streamline processes but also pave the way for future applications across various domains within systems engineering.
The Importance of Requirements Analysis
Requirements analysis is a critical step in systems engineering, serving as the foundation for product quality and precision. By meticulously analyzing system requirements based on INCOSE's criteria, engineers can identify both functional and non-functional needs that drive project success. This process not only clarifies stakeholder expectations but also mitigates risks associated with misinterpretations—especially relevant when integrating generative AI technologies.
Enhancing Product Quality
Effective requirements analysis ensures that all necessary specifications are captured accurately, which directly influences the final product's performance and reliability. In projects like developing RFID-based systems for managing medical equipment, precise requirement classification becomes essential to meet operational standards. Moreover, ongoing evaluation of AI models used in this context helps refine their capabilities to handle complex engineering demands effectively.
Incorporating natural language processing (NLP) and machine learning (ML) techniques into requirements analysis further enhances efficiency by automating classification tasks. However, it remains vital to address potential challenges posed by AI misinterpretations through rigorous testing and validation processes. As technology evolves, so too must our approach to understanding and implementing robust requirements analysis methodologies within systems engineering frameworks.
How AI Transforms Requirements Gathering
AI is revolutionizing requirements gathering in systems engineering by leveraging Natural Language Processing (NLP) and Machine Learning (ML) techniques. These technologies automate the analysis and classification of system requirements, enhancing both product quality and precision. By categorizing requirements into functional or non-functional types, AI helps streamline the development process, reducing human error associated with manual interpretation. However, challenges such as misinterpretations by AI models necessitate rigorous evaluation within engineering contexts to ensure reliability.
Enhancing Efficiency Through Automation
Generative AI tools like ChatGPT and OpenAI's Llama model are pivotal in this transformation. They facilitate rapid processing of vast amounts of data, enabling engineers to focus on higher-level design tasks rather than getting bogged down in minutiae. A notable application includes an RFID-based system for managing medical equipment in operating rooms—demonstrating how effective requirement classification can lead to improved operational efficiency. Continued research aims at refining these models further for better handling of complex engineering demands while emphasizing responsible integration into educational frameworks to prepare future engineers effectively.
Case Studies: AI in Action
Generative AI is revolutionizing systems engineering by automating critical processes such as requirements analysis. A notable case study involves the development of an RFID-based system for managing medical equipment in operating rooms, where natural language processing (NLP) and machine learning (ML) techniques were employed to classify system requirements into functional and non-functional categories. This project demonstrated how AI models can enhance product quality by accurately interpreting complex specifications based on INCOSE's criteria. However, challenges like misinterpretation of requirements necessitate rigorous evaluation of these models within engineering contexts.
Applications of Gaussian Processes
Another significant application highlighted in the research is the use of Gaussian Process Regression for solving inverse problems related to linear partial differential equations (PDEs). By leveraging commutative algebra and implementing algorithms through Macaulay2 software, researchers achieved high accuracy even with noisy data inputs. The method effectively reconstructed wave propagation while addressing local minima issues through sequential training strategies, showcasing both computational efficiency and predictive precision essential for modern engineering tasks.
These case studies underscore not only the transformative potential of AI technologies but also emphasize ongoing research needs to refine these tools further for practical applications across various domains within systems engineering.# Challenges and Limitations of AI Integration
Integrating AI into systems engineering presents several challenges and limitations that must be addressed for successful implementation. One significant issue is the potential for misinterpretation of requirements by AI models, which can lead to inaccurate classifications between functional and non-functional requirements. This misalignment not only affects product quality but also compromises precision in engineering outcomes. Additionally, evaluating the performance of these AI models within specific engineering contexts remains a complex task; traditional metrics may not adequately capture their effectiveness or reliability.
Addressing Misinterpretations
The reliance on Natural Language Processing (NLP) techniques introduces another layer of complexity, as nuances in human language can result in varied interpretations by algorithms. Furthermore, while advancements like Gaussian Process Regression show promise in solving inverse problems efficiently, they are still susceptible to local minima issues—especially prevalent in high-frequency scenarios. As engineers strive to refine these technologies further, it becomes essential to foster responsible integration practices through education and continuous research development initiatives aimed at enhancing model accuracy and applicability across diverse engineering domains.# Future Trends in AI and Systems Engineering
The integration of generative AI into systems engineering is poised to revolutionize the field by automating critical processes such as requirements analysis. By leveraging Natural Language Processing (NLP) and Machine Learning (ML), engineers can enhance product quality through precise classification of functional and non-functional requirements. However, challenges remain, particularly regarding AI misinterpretations that could lead to inaccuracies in system design. The ongoing refinement of AI models will be essential for addressing these issues while ensuring responsible implementation within engineering education. This focus on training future engineers highlights the necessity for a curriculum that incorporates emerging technologies.
Advancements in Computational Techniques
Recent developments have introduced computationally efficient algorithms like Gaussian Process Regression for solving inverse problems related to linear Partial Differential Equations (PDEs). These advancements not only improve accuracy but also optimize performance when reconstructing wave propagation from noisy data. As research progresses, there’s potential for applying these methods to more complex scenarios, including vectorial wave equations and large-scale seismic datasets, which may further enhance predictive capabilities in systems engineering contexts.
In summary, continuous exploration of AI's role alongside mathematical techniques promises significant improvements across various applications within systems engineering while addressing existing limitations effectively.
In conclusion, the integration of AI into systems engineering is poised to revolutionize the field, particularly in requirements analysis. Understanding the foundational aspects of systems engineering and recognizing the critical role that requirements analysis plays are essential for any successful project. AI enhances this process by streamlining data gathering, improving accuracy, and enabling more effective stakeholder engagement through advanced analytics and machine learning algorithms. The case studies presented illustrate tangible benefits realized by organizations that have embraced these technologies, showcasing increased efficiency and reduced time-to-market. However, it is crucial to remain aware of potential challenges such as data privacy concerns and algorithmic biases that can arise with AI implementation. Looking ahead, trends indicate a growing synergy between AI advancements and systems engineering practices, promising even greater innovations on the horizon. Embracing these changes will be vital for professionals aiming to stay competitive in an increasingly complex technological landscape.
FAQs on "Revolutionizing Systems Engineering: The Role of AI in Requirements Analysis"
1. What is systems engineering and why is it important?
Systems engineering is an interdisciplinary field that focuses on the design, integration, and management of complex systems throughout their life cycles. It is important because it ensures that all components of a system work together effectively to meet user needs and project goals.
2. Why is requirements analysis critical in systems engineering?
Requirements analysis is crucial because it defines what a system should accomplish from the user's perspective. It helps identify stakeholder needs, reduces risks associated with misunderstandings or miscommunications, and lays the foundation for successful project execution.
3. How does AI enhance requirements gathering in systems engineering?
AI enhances requirements gathering by automating data collection processes, analyzing large volumes of information quickly, identifying patterns or trends within user feedback, and providing insights that help refine requirements more efficiently than traditional methods.
4. Can you provide examples of how AI has been applied in real-world requirements analysis cases?
Yes! Case studies have shown that organizations use AI tools to analyze customer feedback for software development projects or employ natural language processing (NLP) algorithms to extract relevant information from documents during requirement elicitation phases.
5. What are some challenges associated with integrating AI into requirements analysis?
Challenges include ensuring data quality for accurate results, addressing biases present in training datasets which may affect outcomes, managing resistance to change among team members accustomed to traditional methods, and understanding legal implications related to data privacy when using AI technologies.